Holocene Ice Window Size#
import pyleoclim as pyleo
import matplotlib.pyplot as plt
import numpy as np
from tqdm import tqdm
import ammonyte as amt
import seaborn as sns
import pandas as pd
from pylipd.lipd import LiPD
#We suppress warnings for these notebooks for presentation purposes. Best practice is to not do this though.
import warnings
warnings.filterwarnings('ignore')
color_list = sns.color_palette('colorblind')
lipd_path = './data/8k_ice'
all_files = LiPD()
if __name__=='__main__':
all_files.load_from_dir(lipd_path)
record_names = all_files.get_all_dataset_names()
Directory ./data/8k_ice does not exist
series_list = []
# We specify the indices of interest in each dataframe by hand here
index_dict = {
'GRIP.GRIP.1992' : 'd18O',
'Renland.Johnsen.1992' : 'd18O',
'EDML.Stenni.2010' : 'bagd18O',
'EPICADomeC.Stenni.2010' : 'bagd18O',
'Vostok.Vimeux.2002' : 'temperature',
'GISP2.Grootes.1997' : 'd18O',
'NGRIP.NGRIP.2004' : 'd18O',
'TALDICE.Mezgec.2017' : 'd18O',
}
for record in record_names:
d = LiPD()
d.load(f'{lipd_path}/{record}.lpd')
df = d.get_timeseries_essentials()
row = df[df['paleoData_variableName']==index_dict[record]][df['time_variableName']=='age']
lat = row['geo_meanLat'].to_numpy()[0]
lon = row['geo_meanLon'].to_numpy()[0]
elevation = row['geo_meanElev'].to_numpy()[0]
value = row['paleoData_values'].to_numpy()[0]
value_name = row['paleoData_variableName'].to_numpy()[0]
value_unit = row['paleoData_units'].to_numpy()[0]
time = row['time_values'].to_numpy()[0]
time_unit = row['time_units'].to_numpy()[0]
time_name = row['time_variableName'].to_numpy()[0]
label = row['dataSetName'].to_numpy()[0]
geo_series = pyleo.GeoSeries(time=time,
value=value,
lat=lat,
lon=lon,
elevation=elevation,
time_unit=time_unit,
time_name=time_name,
value_name=value_name,
value_unit=value_unit,
label=label,
archiveType='ice')
series_list.append(geo_series)
geo_ms = pyleo.MultipleGeoSeries(series_list)
ms_list = []
for series in geo_ms.series_list:
if series.lat > 0 or series.label == 'EPICADomeC.Stenni.2010':
series.time_unit = 'Years BP'
ms_list.append(series)
end_time = 10000
ms_ice = pyleo.MultipleSeries([series.slice((0,end_time)) for series in ms_list])
ms_ice.stackplot(colors=color_list[:len(ms_ice.series_list)])
---------------------------------------------------------------------------
ValueError Traceback (most recent call last)
Cell In[7], line 4
1 end_time = 10000
3 ms_ice = pyleo.MultipleSeries([series.slice((0,end_time)) for series in ms_list])
----> 4 ms_ice.stackplot(colors=color_list[:len(ms_ice.series_list)])
File ~/miniconda3/envs/ammonyte/lib/python3.10/site-packages/pyleoclim/core/multipleseries.py:1866, in MultipleSeries.stackplot(self, figsize, savefig_settings, time_unit, xlim, fill_between_alpha, colors, cmap, norm, labels, ylabel_fontsize, spine_lw, grid_lw, label_x_loc, v_shift_factor, linewidth, plot_kwargs)
1863 raise ValueError("The length of the label list should match the number of timeseries to be plotted")
1865 # deal with time units
-> 1866 self = self.convert_time_unit(time_unit=time_unit)
1868 # Deal with plotting arguments
1869 if type(plot_kwargs)==dict:
File ~/miniconda3/envs/ammonyte/lib/python3.10/site-packages/pyleoclim/core/multipleseries.py:268, in MultipleSeries.convert_time_unit(self, time_unit)
266 for i, u in enumerate(unique_units):
267 count_units[i] = units.count(u)
--> 268 time_unit = unique_units[count_units.argmax()]
270 new_ms = self.copy()
271 new_ts_list = []
ValueError: attempt to get argmax of an empty sequence
m = 12
w_size_list = np.arange(10,51,10)
res_dict = {}
lp_dict = {}
for idx,series in enumerate(ms_ice.series_list):
name = series.label
print(f'Analyzing {name}')
for w_size in tqdm(w_size_list):
series_slice = series.slice((0,end_time))
td = amt.TimeEmbeddedSeries(series.slice((0,end_time)),m)
eps = td.find_epsilon(eps=1,target_density=.05,tolerance=.01,verbose=False)
rm = eps['Output']
lp = rm.laplacian_eigenmaps(w_size=w_size,w_incre=4)
if name not in res_dict:
res_dict[name] = []
if name not in lp_dict:
lp_dict[name] = []
lp_dict[name].append(lp)
Analyzing Renland.Johnsen.1992
0%| | 0/5 [00:00<?, ?it/s]/Users/alexjames/Documents/GitHub/Ammonyte/ammonyte/core/rqa_res.py:22: UserWarning: auto_time_params is not specified. Currently default behavior sets this to True. In a future release this will be changed to False.
super().__init__(time,value,time_name,time_unit,value_name,value_unit,label,sort_ts=None)
/Users/alexjames/Documents/GitHub/Ammonyte/ammonyte/core/rqa_res.py:22: UserWarning: No time_name parameter provided. Assuming "Time".
super().__init__(time,value,time_name,time_unit,value_name,value_unit,label,sort_ts=None)
20%|██ | 1/5 [00:00<00:01, 2.39it/s]/Users/alexjames/Documents/GitHub/Ammonyte/ammonyte/core/rqa_res.py:22: UserWarning: auto_time_params is not specified. Currently default behavior sets this to True. In a future release this will be changed to False.
super().__init__(time,value,time_name,time_unit,value_name,value_unit,label,sort_ts=None)
/Users/alexjames/Documents/GitHub/Ammonyte/ammonyte/core/rqa_res.py:22: UserWarning: No time_name parameter provided. Assuming "Time".
super().__init__(time,value,time_name,time_unit,value_name,value_unit,label,sort_ts=None)
40%|████ | 2/5 [00:00<00:01, 2.17it/s]/Users/alexjames/Documents/GitHub/Ammonyte/ammonyte/core/rqa_res.py:22: UserWarning: auto_time_params is not specified. Currently default behavior sets this to True. In a future release this will be changed to False.
super().__init__(time,value,time_name,time_unit,value_name,value_unit,label,sort_ts=None)
/Users/alexjames/Documents/GitHub/Ammonyte/ammonyte/core/rqa_res.py:22: UserWarning: No time_name parameter provided. Assuming "Time".
super().__init__(time,value,time_name,time_unit,value_name,value_unit,label,sort_ts=None)
60%|██████ | 3/5 [00:01<00:01, 1.73it/s]/Users/alexjames/Documents/GitHub/Ammonyte/ammonyte/core/rqa_res.py:22: UserWarning: auto_time_params is not specified. Currently default behavior sets this to True. In a future release this will be changed to False.
super().__init__(time,value,time_name,time_unit,value_name,value_unit,label,sort_ts=None)
/Users/alexjames/Documents/GitHub/Ammonyte/ammonyte/core/rqa_res.py:22: UserWarning: No time_name parameter provided. Assuming "Time".
super().__init__(time,value,time_name,time_unit,value_name,value_unit,label,sort_ts=None)
80%|████████ | 4/5 [00:02<00:00, 1.40it/s]/Users/alexjames/Documents/GitHub/Ammonyte/ammonyte/core/rqa_res.py:22: UserWarning: auto_time_params is not specified. Currently default behavior sets this to True. In a future release this will be changed to False.
super().__init__(time,value,time_name,time_unit,value_name,value_unit,label,sort_ts=None)
/Users/alexjames/Documents/GitHub/Ammonyte/ammonyte/core/rqa_res.py:22: UserWarning: No time_name parameter provided. Assuming "Time".
super().__init__(time,value,time_name,time_unit,value_name,value_unit,label,sort_ts=None)
100%|██████████| 5/5 [00:03<00:00, 1.37it/s]
Analyzing EPICADomeC.Stenni.2010
0%| | 0/5 [00:00<?, ?it/s]/Users/alexjames/Documents/GitHub/Ammonyte/ammonyte/core/rqa_res.py:22: UserWarning: auto_time_params is not specified. Currently default behavior sets this to True. In a future release this will be changed to False.
super().__init__(time,value,time_name,time_unit,value_name,value_unit,label,sort_ts=None)
/Users/alexjames/Documents/GitHub/Ammonyte/ammonyte/core/rqa_res.py:22: UserWarning: No time_name parameter provided. Assuming "Time".
super().__init__(time,value,time_name,time_unit,value_name,value_unit,label,sort_ts=None)
20%|██ | 1/5 [00:00<00:01, 2.40it/s]/Users/alexjames/Documents/GitHub/Ammonyte/ammonyte/core/rqa_res.py:22: UserWarning: auto_time_params is not specified. Currently default behavior sets this to True. In a future release this will be changed to False.
super().__init__(time,value,time_name,time_unit,value_name,value_unit,label,sort_ts=None)
/Users/alexjames/Documents/GitHub/Ammonyte/ammonyte/core/rqa_res.py:22: UserWarning: No time_name parameter provided. Assuming "Time".
super().__init__(time,value,time_name,time_unit,value_name,value_unit,label,sort_ts=None)
40%|████ | 2/5 [00:01<00:01, 1.91it/s]/Users/alexjames/Documents/GitHub/Ammonyte/ammonyte/core/rqa_res.py:22: UserWarning: auto_time_params is not specified. Currently default behavior sets this to True. In a future release this will be changed to False.
super().__init__(time,value,time_name,time_unit,value_name,value_unit,label,sort_ts=None)
/Users/alexjames/Documents/GitHub/Ammonyte/ammonyte/core/rqa_res.py:22: UserWarning: No time_name parameter provided. Assuming "Time".
super().__init__(time,value,time_name,time_unit,value_name,value_unit,label,sort_ts=None)
60%|██████ | 3/5 [00:01<00:01, 1.51it/s]/Users/alexjames/Documents/GitHub/Ammonyte/ammonyte/core/rqa_res.py:22: UserWarning: auto_time_params is not specified. Currently default behavior sets this to True. In a future release this will be changed to False.
super().__init__(time,value,time_name,time_unit,value_name,value_unit,label,sort_ts=None)
/Users/alexjames/Documents/GitHub/Ammonyte/ammonyte/core/rqa_res.py:22: UserWarning: No time_name parameter provided. Assuming "Time".
super().__init__(time,value,time_name,time_unit,value_name,value_unit,label,sort_ts=None)
80%|████████ | 4/5 [00:03<00:01, 1.03s/it]/Users/alexjames/Documents/GitHub/Ammonyte/ammonyte/core/rqa_res.py:22: UserWarning: auto_time_params is not specified. Currently default behavior sets this to True. In a future release this will be changed to False.
super().__init__(time,value,time_name,time_unit,value_name,value_unit,label,sort_ts=None)
/Users/alexjames/Documents/GitHub/Ammonyte/ammonyte/core/rqa_res.py:22: UserWarning: No time_name parameter provided. Assuming "Time".
super().__init__(time,value,time_name,time_unit,value_name,value_unit,label,sort_ts=None)
100%|██████████| 5/5 [00:05<00:00, 1.02s/it]
Analyzing GISP2.Grootes.1997
0%| | 0/5 [00:00<?, ?it/s]/Users/alexjames/Documents/GitHub/Ammonyte/ammonyte/core/rqa_res.py:22: UserWarning: auto_time_params is not specified. Currently default behavior sets this to True. In a future release this will be changed to False.
super().__init__(time,value,time_name,time_unit,value_name,value_unit,label,sort_ts=None)
/Users/alexjames/Documents/GitHub/Ammonyte/ammonyte/core/rqa_res.py:22: UserWarning: No time_name parameter provided. Assuming "Time".
super().__init__(time,value,time_name,time_unit,value_name,value_unit,label,sort_ts=None)
20%|██ | 1/5 [00:00<00:03, 1.25it/s]/Users/alexjames/Documents/GitHub/Ammonyte/ammonyte/core/rqa_res.py:22: UserWarning: auto_time_params is not specified. Currently default behavior sets this to True. In a future release this will be changed to False.
super().__init__(time,value,time_name,time_unit,value_name,value_unit,label,sort_ts=None)
/Users/alexjames/Documents/GitHub/Ammonyte/ammonyte/core/rqa_res.py:22: UserWarning: No time_name parameter provided. Assuming "Time".
super().__init__(time,value,time_name,time_unit,value_name,value_unit,label,sort_ts=None)
40%|████ | 2/5 [00:02<00:03, 1.17s/it]/Users/alexjames/Documents/GitHub/Ammonyte/ammonyte/core/rqa_res.py:22: UserWarning: auto_time_params is not specified. Currently default behavior sets this to True. In a future release this will be changed to False.
super().__init__(time,value,time_name,time_unit,value_name,value_unit,label,sort_ts=None)
/Users/alexjames/Documents/GitHub/Ammonyte/ammonyte/core/rqa_res.py:22: UserWarning: No time_name parameter provided. Assuming "Time".
super().__init__(time,value,time_name,time_unit,value_name,value_unit,label,sort_ts=None)
60%|██████ | 3/5 [00:04<00:03, 1.62s/it]/Users/alexjames/Documents/GitHub/Ammonyte/ammonyte/core/rqa_res.py:22: UserWarning: auto_time_params is not specified. Currently default behavior sets this to True. In a future release this will be changed to False.
super().__init__(time,value,time_name,time_unit,value_name,value_unit,label,sort_ts=None)
/Users/alexjames/Documents/GitHub/Ammonyte/ammonyte/core/rqa_res.py:22: UserWarning: No time_name parameter provided. Assuming "Time".
super().__init__(time,value,time_name,time_unit,value_name,value_unit,label,sort_ts=None)
80%|████████ | 4/5 [00:07<00:02, 2.06s/it]/Users/alexjames/Documents/GitHub/Ammonyte/ammonyte/core/rqa_res.py:22: UserWarning: auto_time_params is not specified. Currently default behavior sets this to True. In a future release this will be changed to False.
super().__init__(time,value,time_name,time_unit,value_name,value_unit,label,sort_ts=None)
/Users/alexjames/Documents/GitHub/Ammonyte/ammonyte/core/rqa_res.py:22: UserWarning: No time_name parameter provided. Assuming "Time".
super().__init__(time,value,time_name,time_unit,value_name,value_unit,label,sort_ts=None)
100%|██████████| 5/5 [00:10<00:00, 2.20s/it]
Analyzing GRIP.GRIP.1992
0%| | 0/5 [00:00<?, ?it/s]/Users/alexjames/Documents/GitHub/Ammonyte/ammonyte/core/rqa_res.py:22: UserWarning: auto_time_params is not specified. Currently default behavior sets this to True. In a future release this will be changed to False.
super().__init__(time,value,time_name,time_unit,value_name,value_unit,label,sort_ts=None)
/Users/alexjames/Documents/GitHub/Ammonyte/ammonyte/core/rqa_res.py:22: UserWarning: No time_name parameter provided. Assuming "Time".
super().__init__(time,value,time_name,time_unit,value_name,value_unit,label,sort_ts=None)
20%|██ | 1/5 [00:01<00:07, 1.95s/it]/Users/alexjames/Documents/GitHub/Ammonyte/ammonyte/core/rqa_res.py:22: UserWarning: auto_time_params is not specified. Currently default behavior sets this to True. In a future release this will be changed to False.
super().__init__(time,value,time_name,time_unit,value_name,value_unit,label,sort_ts=None)
/Users/alexjames/Documents/GitHub/Ammonyte/ammonyte/core/rqa_res.py:22: UserWarning: No time_name parameter provided. Assuming "Time".
super().__init__(time,value,time_name,time_unit,value_name,value_unit,label,sort_ts=None)
40%|████ | 2/5 [00:05<00:07, 2.64s/it]/Users/alexjames/Documents/GitHub/Ammonyte/ammonyte/core/rqa_res.py:22: UserWarning: auto_time_params is not specified. Currently default behavior sets this to True. In a future release this will be changed to False.
super().__init__(time,value,time_name,time_unit,value_name,value_unit,label,sort_ts=None)
/Users/alexjames/Documents/GitHub/Ammonyte/ammonyte/core/rqa_res.py:22: UserWarning: No time_name parameter provided. Assuming "Time".
super().__init__(time,value,time_name,time_unit,value_name,value_unit,label,sort_ts=None)
60%|██████ | 3/5 [00:10<00:07, 3.77s/it]/Users/alexjames/Documents/GitHub/Ammonyte/ammonyte/core/rqa_res.py:22: UserWarning: auto_time_params is not specified. Currently default behavior sets this to True. In a future release this will be changed to False.
super().__init__(time,value,time_name,time_unit,value_name,value_unit,label,sort_ts=None)
/Users/alexjames/Documents/GitHub/Ammonyte/ammonyte/core/rqa_res.py:22: UserWarning: No time_name parameter provided. Assuming "Time".
super().__init__(time,value,time_name,time_unit,value_name,value_unit,label,sort_ts=None)
80%|████████ | 4/5 [00:17<00:05, 5.12s/it]/Users/alexjames/Documents/GitHub/Ammonyte/ammonyte/core/rqa_res.py:22: UserWarning: auto_time_params is not specified. Currently default behavior sets this to True. In a future release this will be changed to False.
super().__init__(time,value,time_name,time_unit,value_name,value_unit,label,sort_ts=None)
/Users/alexjames/Documents/GitHub/Ammonyte/ammonyte/core/rqa_res.py:22: UserWarning: No time_name parameter provided. Assuming "Time".
super().__init__(time,value,time_name,time_unit,value_name,value_unit,label,sort_ts=None)
100%|██████████| 5/5 [00:25<00:00, 5.17s/it]
Analyzing NGRIP.NGRIP.2004
0%| | 0/5 [00:00<?, ?it/s]/Users/alexjames/Documents/GitHub/Ammonyte/ammonyte/core/rqa_res.py:22: UserWarning: auto_time_params is not specified. Currently default behavior sets this to True. In a future release this will be changed to False.
super().__init__(time,value,time_name,time_unit,value_name,value_unit,label,sort_ts=None)
/Users/alexjames/Documents/GitHub/Ammonyte/ammonyte/core/rqa_res.py:22: UserWarning: No time_name parameter provided. Assuming "Time".
super().__init__(time,value,time_name,time_unit,value_name,value_unit,label,sort_ts=None)
20%|██ | 1/5 [00:00<00:01, 2.92it/s]/Users/alexjames/Documents/GitHub/Ammonyte/ammonyte/core/rqa_res.py:22: UserWarning: auto_time_params is not specified. Currently default behavior sets this to True. In a future release this will be changed to False.
super().__init__(time,value,time_name,time_unit,value_name,value_unit,label,sort_ts=None)
/Users/alexjames/Documents/GitHub/Ammonyte/ammonyte/core/rqa_res.py:22: UserWarning: No time_name parameter provided. Assuming "Time".
super().__init__(time,value,time_name,time_unit,value_name,value_unit,label,sort_ts=None)
40%|████ | 2/5 [00:00<00:01, 2.13it/s]/Users/alexjames/Documents/GitHub/Ammonyte/ammonyte/core/rqa_res.py:22: UserWarning: auto_time_params is not specified. Currently default behavior sets this to True. In a future release this will be changed to False.
super().__init__(time,value,time_name,time_unit,value_name,value_unit,label,sort_ts=None)
/Users/alexjames/Documents/GitHub/Ammonyte/ammonyte/core/rqa_res.py:22: UserWarning: No time_name parameter provided. Assuming "Time".
super().__init__(time,value,time_name,time_unit,value_name,value_unit,label,sort_ts=None)
60%|██████ | 3/5 [00:01<00:01, 1.60it/s]/Users/alexjames/Documents/GitHub/Ammonyte/ammonyte/core/rqa_res.py:22: UserWarning: auto_time_params is not specified. Currently default behavior sets this to True. In a future release this will be changed to False.
super().__init__(time,value,time_name,time_unit,value_name,value_unit,label,sort_ts=None)
/Users/alexjames/Documents/GitHub/Ammonyte/ammonyte/core/rqa_res.py:22: UserWarning: No time_name parameter provided. Assuming "Time".
super().__init__(time,value,time_name,time_unit,value_name,value_unit,label,sort_ts=None)
80%|████████ | 4/5 [00:02<00:00, 1.24it/s]/Users/alexjames/Documents/GitHub/Ammonyte/ammonyte/core/rqa_res.py:22: UserWarning: auto_time_params is not specified. Currently default behavior sets this to True. In a future release this will be changed to False.
super().__init__(time,value,time_name,time_unit,value_name,value_unit,label,sort_ts=None)
/Users/alexjames/Documents/GitHub/Ammonyte/ammonyte/core/rqa_res.py:22: UserWarning: No time_name parameter provided. Assuming "Time".
super().__init__(time,value,time_name,time_unit,value_name,value_unit,label,sort_ts=None)
100%|██████████| 5/5 [00:04<00:00, 1.24it/s]
name = ms_list[0].label
fig,ax = plt.subplots(nrows=len(lp_dict[name]),figsize=(10,4*len(w_size_list)),sharex=True)
fig.subplots_adjust(hspace=.5)
axes = ax.ravel()
for idx,series in tqdm(enumerate(lp_dict[name])):
series.confidence_fill_plot(ax=axes[idx],title=f'W_size = {w_size_list[idx]}')
axes[idx].set_ylabel('FI')
if idx < len(axes)-1:
axes[idx].set_xlabel('')
axes[idx].get_legend().remove()
fig.suptitle(name)
5it [00:11, 2.21s/it]
Text(0.5, 0.98, 'Renland.Johnsen.1992')
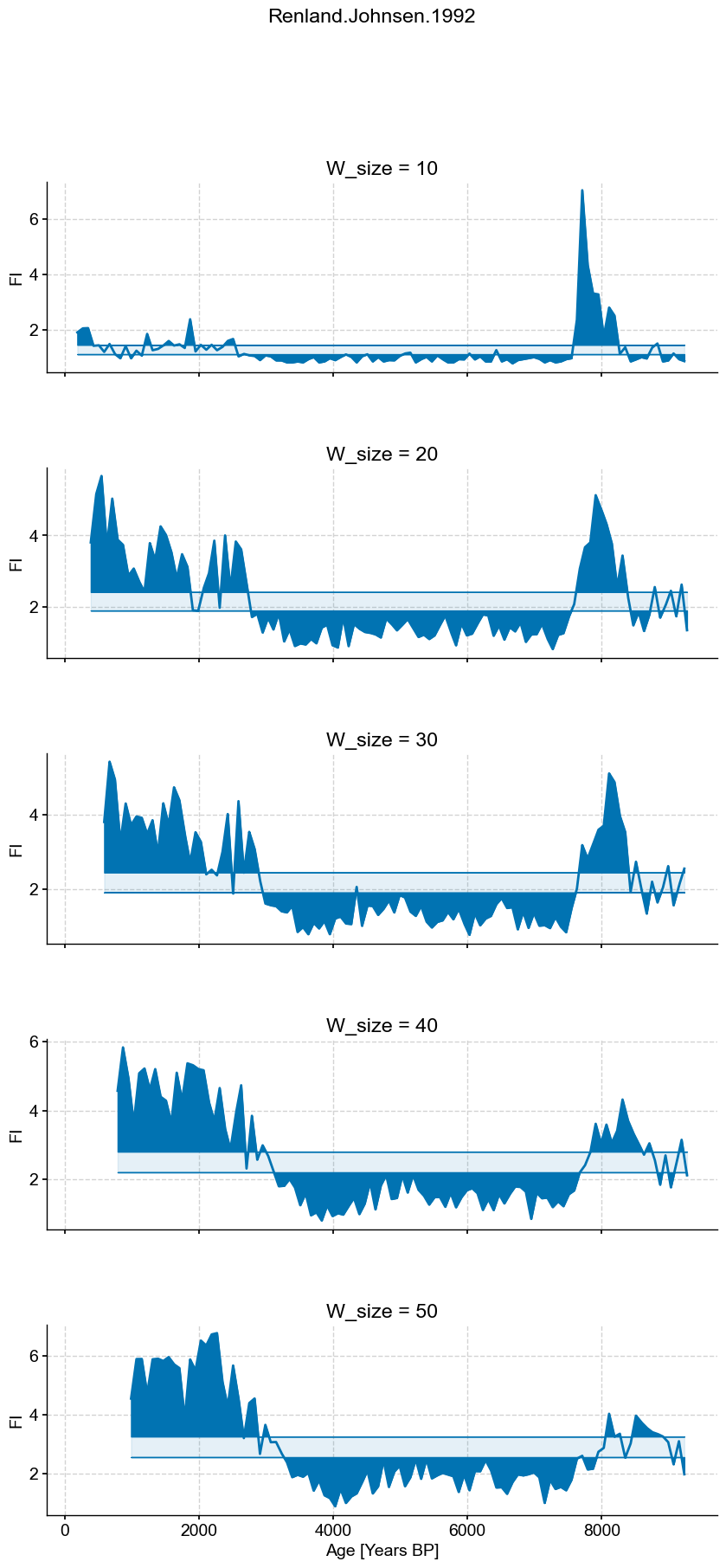
name = ms_list[1].label
fig,ax = plt.subplots(nrows=len(lp_dict[name]),figsize=(10,4*len(w_size_list)),sharex=True)
fig.subplots_adjust(hspace=.5)
axes = ax.ravel()
for idx,series in tqdm(enumerate(lp_dict[name])):
series.confidence_fill_plot(ax=axes[idx],title=f'W_size = {w_size_list[idx]}')
axes[idx].set_ylabel('FI')
if idx < len(axes)-1:
axes[idx].set_xlabel('')
axes[idx].get_legend().remove()
fig.suptitle(name)
0it [00:00, ?it/s]
5it [00:11, 2.23s/it]
Text(0.5, 0.98, 'EPICADomeC.Stenni.2010')
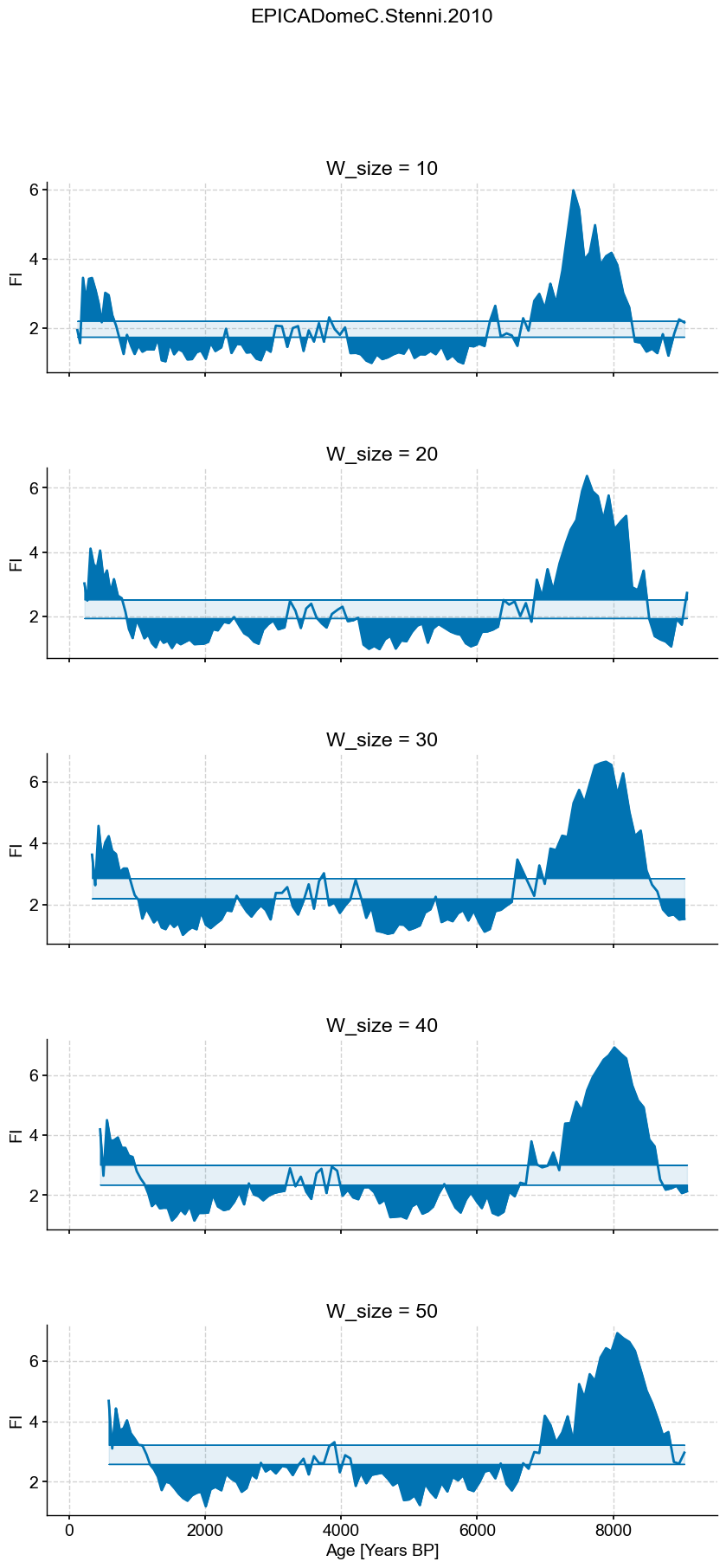
name = ms_list[2].label
fig,ax = plt.subplots(nrows=len(lp_dict[name]),figsize=(10,4*len(w_size_list)),sharex=True)
fig.subplots_adjust(hspace=.5)
axes = ax.ravel()
for idx,series in tqdm(enumerate(lp_dict[name])):
series.confidence_fill_plot(ax=axes[idx],title=f'W_size = {w_size_list[idx]}')
axes[idx].set_ylabel('FI')
if idx < len(axes)-1:
axes[idx].set_xlabel('')
axes[idx].get_legend().remove()
fig.suptitle(name)
0it [00:00, ?it/s]
5it [00:11, 2.30s/it]
Text(0.5, 0.98, 'GISP2.Grootes.1997')
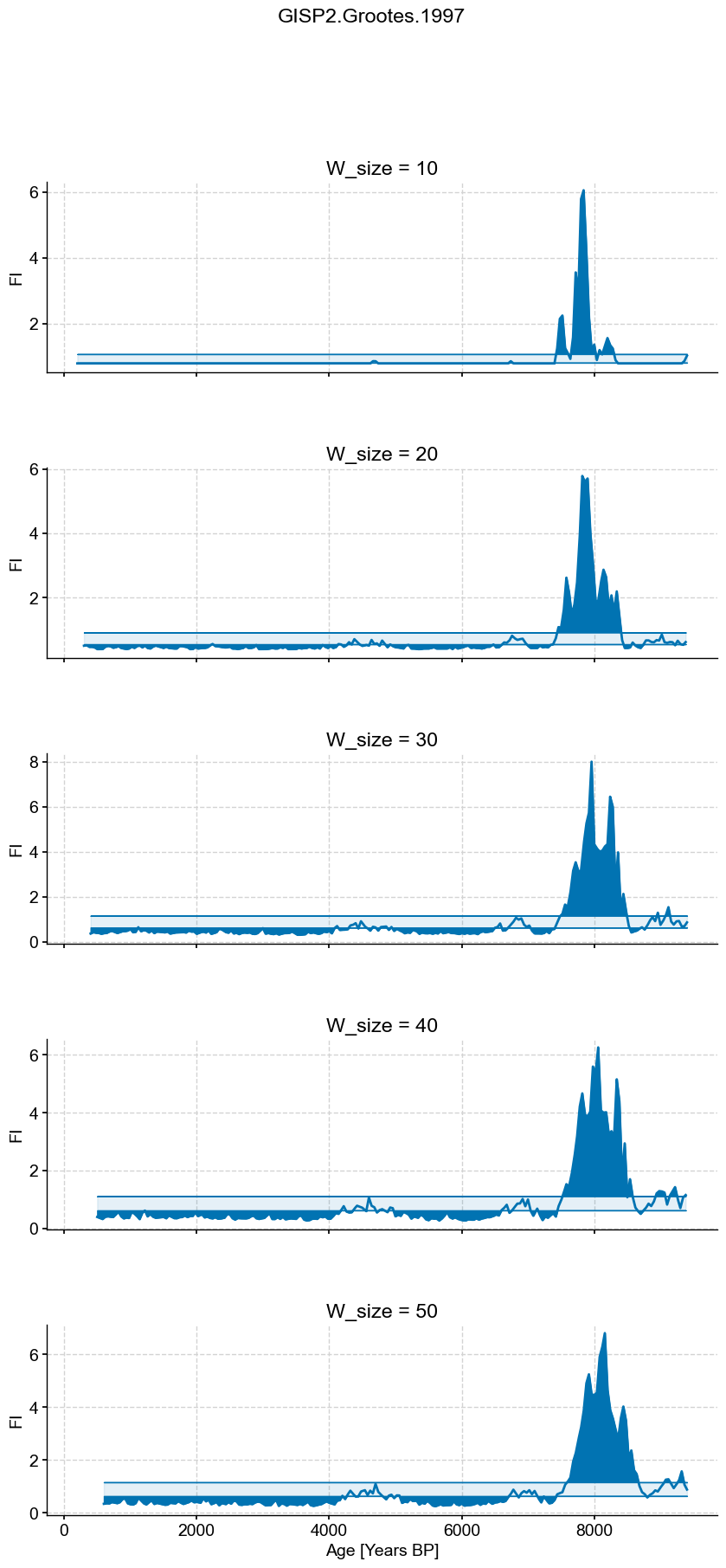
name = ms_list[3].label
fig,ax = plt.subplots(nrows=len(lp_dict[name]),figsize=(10,4*len(w_size_list)),sharex=True)
fig.subplots_adjust(hspace=.5)
axes = ax.ravel()
for idx,series in tqdm(enumerate(lp_dict[name])):
series.confidence_fill_plot(ax=axes[idx],title=f'W_size = {w_size_list[idx]}')
axes[idx].set_ylabel('FI')
if idx < len(axes)-1:
axes[idx].set_xlabel('')
axes[idx].get_legend().remove()
fig.suptitle(name)
0it [00:00, ?it/s]
5it [00:12, 2.50s/it]
Text(0.5, 0.98, 'GRIP.GRIP.1992')
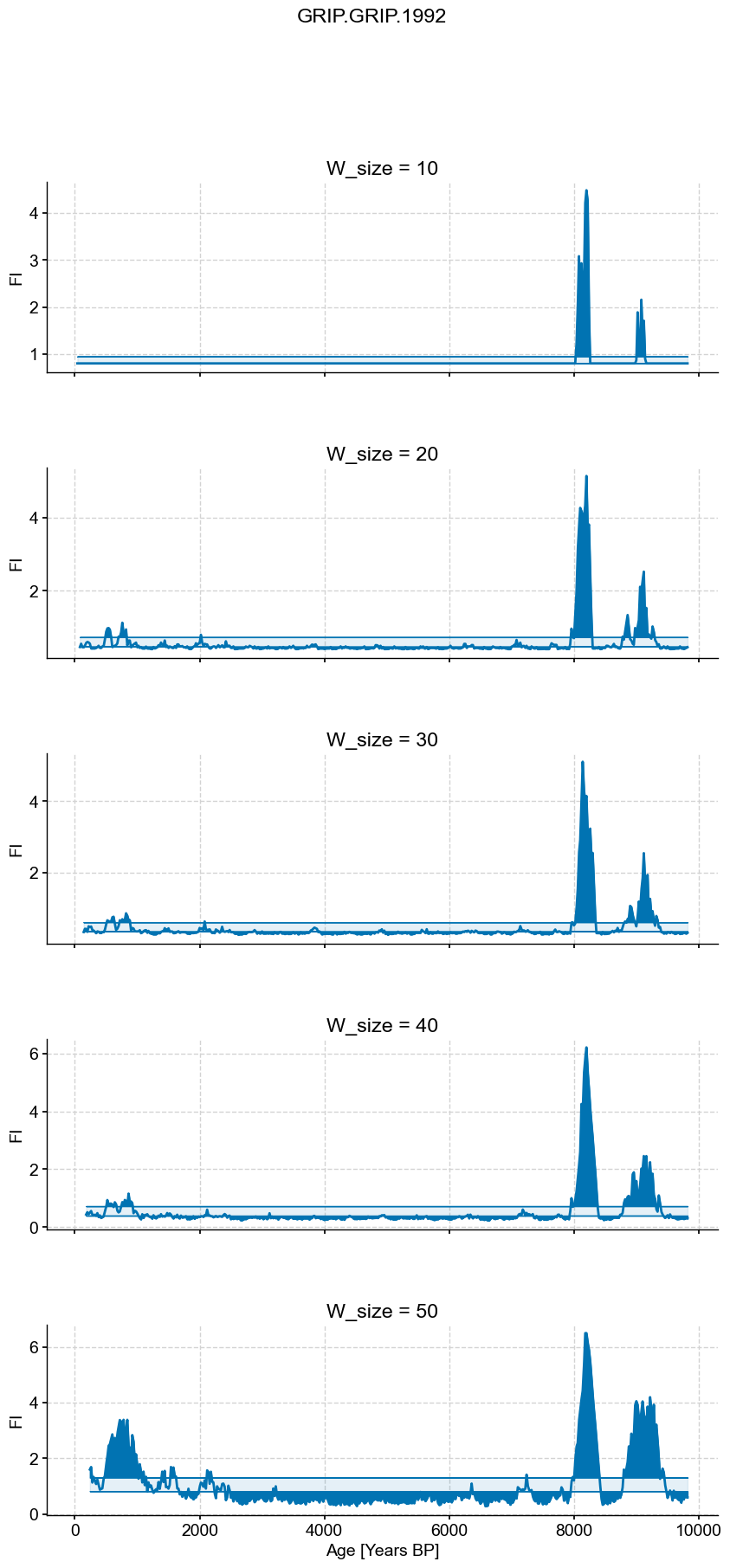
name = ms_list[4].label
fig,ax = plt.subplots(nrows=len(lp_dict[name]),figsize=(10,4*len(w_size_list)),sharex=True)
fig.subplots_adjust(hspace=.5)
axes = ax.ravel()
for idx,series in tqdm(enumerate(lp_dict[name])):
series.confidence_fill_plot(ax=axes[idx],title=f'W_size = {w_size_list[idx]}')
axes[idx].set_ylabel('FI')
if idx < len(axes)-1:
axes[idx].set_xlabel('')
axes[idx].get_legend().remove()
fig.suptitle(name)
5it [00:10, 2.10s/it]
Text(0.5, 0.98, 'NGRIP.NGRIP.2004')
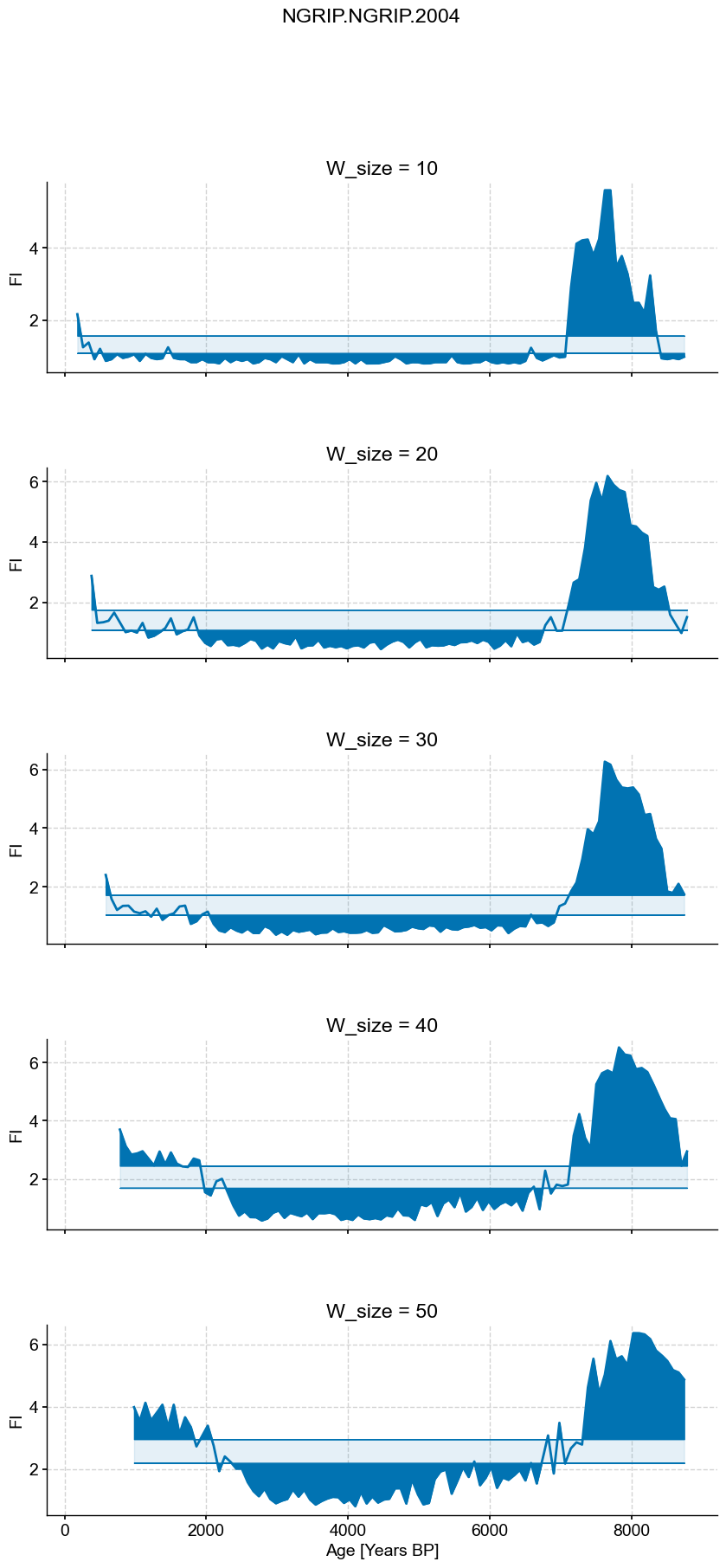